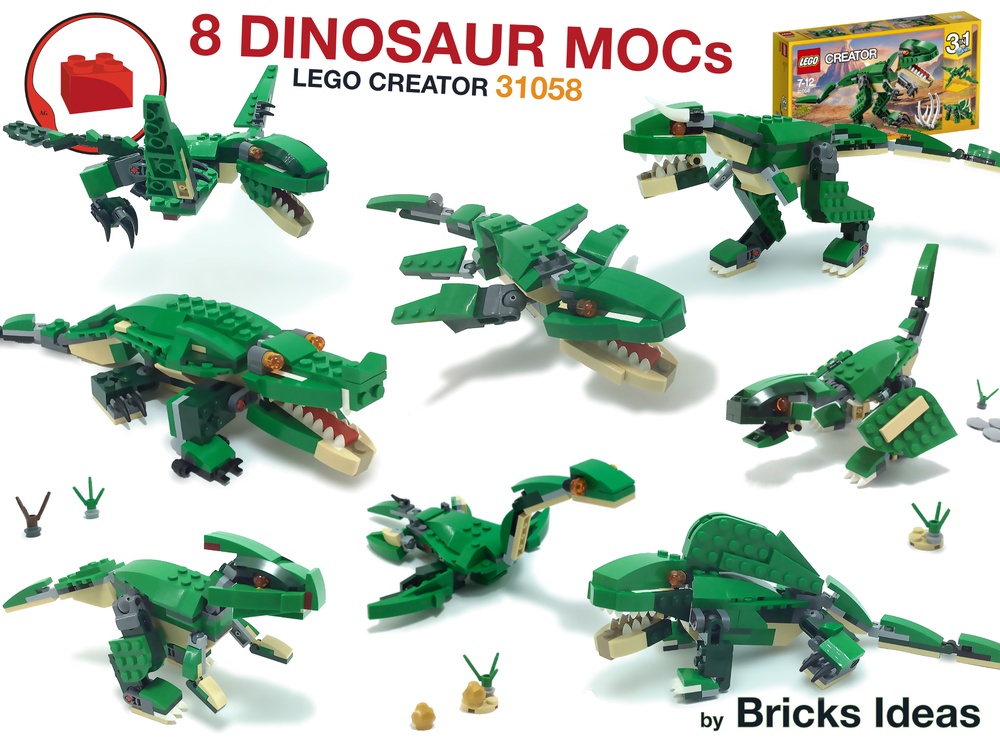
LEGO MOC 8 in 1 - Dinosaurs - Lego Creator 31058 MOCs by Bricks Ideas | Rebrickable - Build with LEGO

LEGO Creator Mighty Dinosaurs 3 in 1 31058 T Rex Triceratops Pterodactyl Set New 673419266482 | eBay

Amazon.com: GARUNK 6 in 1 Mighty Dinosaurs Building Blocks Set, Build It Yourself Dinosaur Set, Create a Ceratosaurus, Pterosaur, Diplodocus, Triceratops, Allosaurus, Raptor, for Kids (673 Pieces) : Toys & Games
LEGO Creators 3-in-1 Mighty Dinosaurs SECRET FOURTH DINOSAUR! | Today on Bricktopia, we speed build and review LEGO Creators 3-in-1 Mighty Dinosaurs set 31058, and we found a SECRET DINOSAUR to build!

Amazon.com: LEGO Creator Mighty Dinosaur Toy 31058, 3 in 1 Model, T. rex, Triceratops and Pterodactyl Dinosaur Figures, Gifts for 7-12 Year Old Boys & Girls : Toys & Games
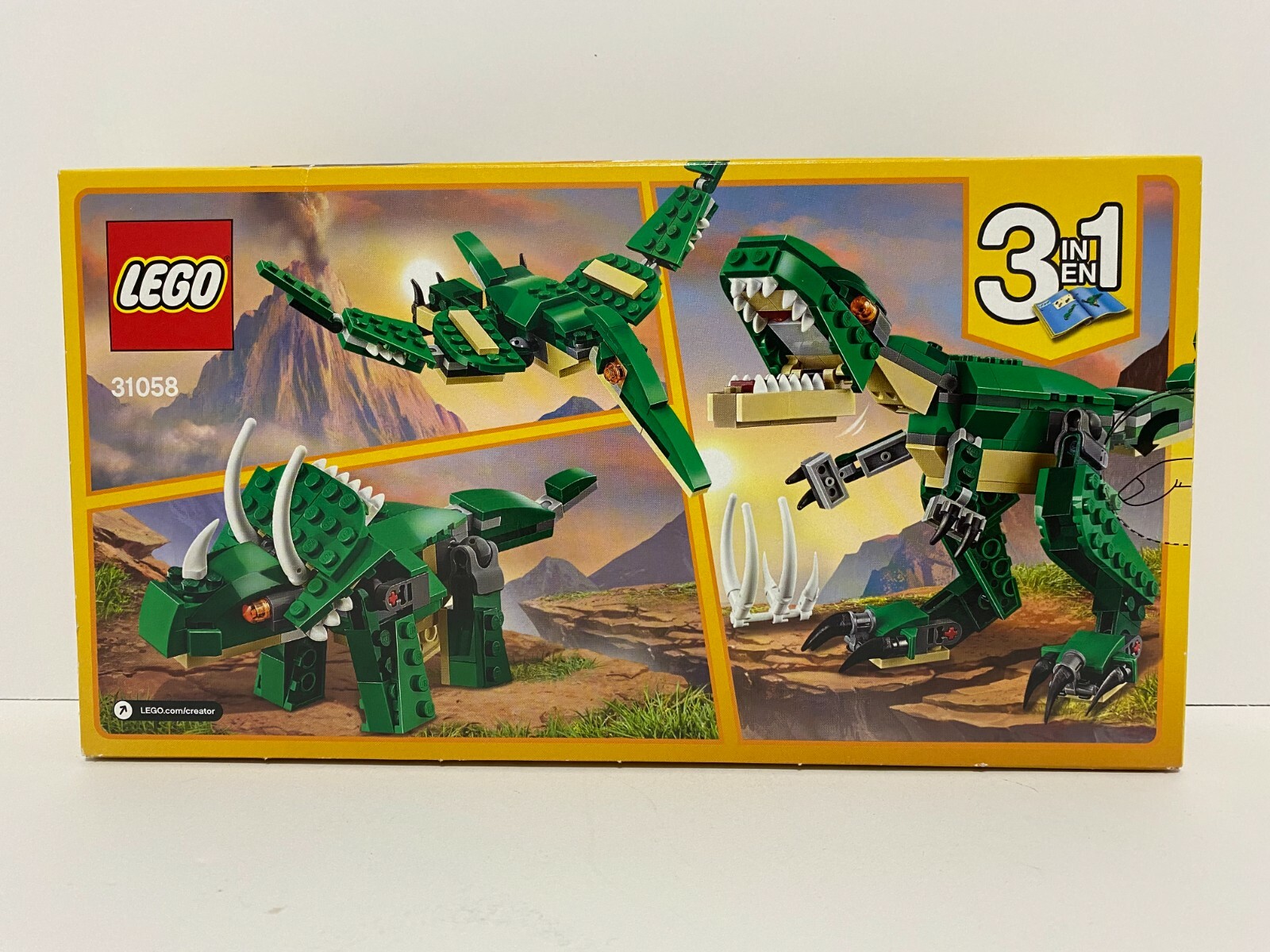
LEGO Creator Mighty Dinosaurs 174 Pcs 31058 3 in 1 T-Rex Pterodactyl Triceratops 673419266482 | eBay

Amazon.com: Lego 31058 Creator Mighty Dinosaurs Toy, 3 in 1 Model, Triceratops and Pterodactyl Dinosaur Figures, Modular Building System : Creator Mighty Dinosaurs: Toys & Games
